5 LLMs, 1vs1 matches to produce the most convincing argumentation in favor or against a random motion. Oh, and also the debate judge is an LLM :)
1. Introduction
Large Language Models (LLMs) have revolutionized our everyday life since the launch of ChatGPT in november 2022: OpenAI’s LLM-powered chat application gained one million users in 5 days and, in October 2024, after almost two years from each launch, reached 3.7 billions of visit in a single month, putting it 11th on the shortlists of the most visited websites.
This broad adoption of text-generating Artificial Intelligence (AI) is also reflected in the skyrocketing number of LLM releases by numerous companies: while OpenAI, Anthropic or other big AI companies build mostly closed-source products, these new models, available mainly on HuggingFace Hub, are mostly open-weight or open-source (for an explanation of the difference see this article). Leading the open AI revolution are companies like Meta, Qwen (by Alibaba), Huggingface (HF), Microsoft and many others.
Open models are progressively getting closer in performance to their closed-source counterparts, matching them in many tasks like coding or, with the latest releases, reasoning.
With open LLMs becoming better at complex jobs, one of the fields they can be tested on is debating. There has been some research already on the topic, whose most relevant contributions can be summarized with:
- Agent4Debate (Zhang et al., 2024): a collaborative framework leveraging a Searcher, an Analyzer, a Writer and a Reviewer to mimic human behavior for debate preparation and execution. Evaluated against human and other baseline models, Agent4Debate demonstrated human-comparable capabilities
- Debatrix (Liang et al., 2024): a comprehensive LLM judge for multi-turn debate settings
- Debates used to evaluate the performance of LLMs (Moniri et al., 2024): an automated evaluation framework based on debates among LLMs which are judged by another LLM. This helps in scaling the benchmarking of Language models outside domain-specific knowledge or fixed test sets
- DebateBrawl (Aryan, 2024): a platform that, integrating genetic algorithms and game theory strategies with LLM reasoning and text generation capabilities, provides the users with an interactive debate experience by crafting coherent and poignant arguments.
In this blog post, we will propose a Debate Championship among five state-of-the-art open models available through HuggingFace Inference API.
2. Materials and Methods
2a. General Structure of the Tournament
The tournament is structured with the so called “Italian” formula, meaning that all participants play with all the others. There is no “home and away games” schema: every participant plays with each of the other ones only once. A model earns one point by winning a game, whereas it does not earn any (but it does not lose any as well) when losing a game.
Each tournament round is one-shot, meaning that each participant has only one possibility to generate a 150-250 words argument, that will be then judged by an external LLM.
This first tournament consists of 5 LLMs as debaters:
And two as judges:
2b. Data Collection and Processing
Code reference: DebateChampionshipLLMs.ipynb
The motions which were used to prompt the debate matches were extracted from kokhayas/english-debate-motions-utds
dataset on HuggingFace.
1,000 of them were then randomly sampled from the 10,000+ set of motions contained in the original dataset, and a random motion was selected for each debate round.
from datasets import load_dataset
# download the dataset from HF hub
dts = load_dataset("kokhayas/english-debate-motions-utds")
dtsdct = dts["train"]
import random as r
# sample 1000 motions from the original dataset
motions = dtsdct["motion"]
motions2use = []
numbers = []
j = 0
while j < 1000:
n = r.randint(0,10000)
if n not in numbers:
numbers.append(n)
if motions[n].lower().startswith("th"):
motions2use.append(motions[n])
j+=1
else:
continue
else:
continue
2c. Building and Running the Tournament
Code reference: DebateChampionshipLLMs.ipynb
We approached building the tournament by:
- decomposing it into its atomic parts, the “building blocks” (defining how debaters and judges generate their answers)
- scaling to creating the structure of one round (debater 1 -> debater 2 -> judge)
- defining the entire tournament as a loop of rounds, with debate data collection and points tracking (for the final ranking)
The code to create the building blocks of the debate tournament is the following:
from huggingface_hub import InferenceClient
from google.colab import userdata
# create an HF client for inference
hf_token = userdata.get('HF_TOKEN_INFERENCE')
client = InferenceClient(api_key=hf_token)
# define a function for the debaters to produce their argument
def debate_inference(model, prompt):
messages = [
{"role": "system", "content": "You are skilled in competitive debate. You produce arguments that strictly adhere to the position you are required to take by the prompts you are proposed with"},
{"role": "user", "content": prompt}
]
completion = client.chat.completions.create(
model=model,
messages=messages,
temperature=0.5,
max_tokens=2048,
top_p=0.7
)
return completion.choices[0].message.content
# define a function for the judges to produce their verdict
def judge_inference(model, motion, essay1, essay2):
messages = [
{"role": "system", "content": "You are a judge, based on the motion, the argumentation in favor of it and the argumentation against it, you should produce a JSON string that contains the following fields:\n\n- winner (str): can take only FAVOR or AGAINST as values, based on who you think the winner is\n- reasons (str): the reasons why you chose the winner. OUTPUT ONLY THE JSON STRING AS: '''\n\n```json\n{\"winner\": 'FAVOR'|'AGAINST', \"reasons\": 'Reasons why you chose the winner'}\n```\n\n'''"},
{"role": "user", "content": "MOTION:\n"+motion},
{"role": "user", "content": "ARGUMENT IN FAVOR:\n"+essay1},
{"role": "user", "content": "ARGUMENT AGAINST:\n"+essay2},
{"role": "user", "content": "Who is the winner? OUTPUT ONLY THE JSON STRING AS: '''\n\n```json\n{\"winner\": 'FAVOR'|'AGAINST', \"reasons\": 'Reasons why you chose the winner'}\n```\n\n'''"}
]
completion = client.chat.completions.create(
model=model,
messages=messages,
temperature=0,
max_tokens=2048,
top_p=0.7
)
return completion.choices[0].message.content
# define a tournament round
def tournament_round(model1, model2, judge, motion):
prompt1 = "Produce an essay of maximum 150 words in favor of this motion: " + motion
prompt2 = "Produce an essay of maximum 150 words against this motion: " + motion
essay1 = debate_inference(model1, prompt1)
essay2 = debate_inference(model2, prompt2)
winner_answer = judge_inference(judge, motion, essay1, essay2)
return essay1, essay2, winner_answer
For the tournament itself to be run, we add the following features to the backbone structure:
- Point tracking
- Debate data collection
- winner and reasons for winner’s choice extraction from the judge’s answer
The last point is especially painful, since the judge’s answer can come in various formats even if the system instructions are very clear on how to structure it, so we decided to tackle the challenge posed by the variability of the output by adding a output parser LLM. This output parser LLM is gpt-4o-mini
, that is wrapped into Langchain OpenAI chat class (ChatOpenaAI
), and linked to a Pydantic schema for structured output generation:
from google.colab import userdata
import os
# set OpenAI API key as an environment variable
a = userdata.get('OPENAI_API_KEY')
os.environ["OPENAI_API_KEY"] = a
from langchain_openai import ChatOpenAI
from langchain_core.prompts import ChatPromptTemplate
from langchain_core.pydantic_v1 import BaseModel, Field
# generate a chat prompt template with Langchain, to wrap your system instructions for the model
GPT_MODEL = "gpt-4o-mini"
llm = ChatOpenAI(temperature=0, model=GPT_MODEL)
system_prompt = ChatPromptTemplate.from_messages(
[
(
"system",
"""You are a helpful assistant. Your job is to restructure the virdict from a debate competition so that it follows this structure:
- winner: the winner, as reported by the virdict
- reasons: reasons for the choice of the winner
Strictly follow the virdict you are provided with, do not add/make up any information."""),
("human", "{message}"),
]
)
from pydantic import BaseModel, Field
# create a Pydantic BaseModel for structured output generation
class Verdict(BaseModel):
"""Structure of the output of a debate competition verdict"""
winner: str = Field(description="The winner, as reported by the verdict")
reasons: str = Field(description="Reasons for the choice of the winner")
# define an inference-ready system instructions+LLM+structured output parser
chain = system_prompt | llm.with_structured_output(Verdict)
Now we can run the tournament:
import time
# define points tracker
modelpoints = {judges[i]: {model: 0 for model in models} for i in range(len(judges))}
# define data collector
motions2args2winner2reasons = {"motions": [], "judge": [], "favor_model": [], "favor_arg": [], "against_model": [], "against_arg": [], "winner": [], "reasons": [], "total_time": []}
judge_counter = 0
for judge in judges:
judge_counter+=1
pairs = []
counter = 0
for i in range(len(models)):
for j in range(len(models)):
# only make two models play with each other if they have not met before
if i!=j and (i,j) not in pairs and (j,i) not in pairs:
counter+=1
pairs.append((i,j))
motion = r.choice(motions2use)
favoragainst = {"favor": models[i], "against": models[j]}
s = time.time()
favor_arg, against_arg, winner_json = tournament_round(models[i], models[j], judge, motion)
e = time.time()
# add debate data to data collector
motions2args2winner2reasons["total_time"].append(e-s)
motions2args2winner2reasons["judge"].append(judge)
motions2args2winner2reasons["motions"].append(motion)
motions2args2winner2reasons["favor_model"].append(favoragainst["favor"])
motions2args2winner2reasons["favor_arg"].append(favor_arg)
motions2args2winner2reasons["against_model"].append(favoragainst["against"])
motions2args2winner2reasons["against_arg"].append(against_arg)
virdict = chain.invoke({"message": winner_json})
reasons = virdict.reasons
winner = virdict.winner
winner_model = favoragainst[winner.lower()]
motions2args2winner2reasons["winner"].append(winner_model)
motions2args2winner2reasons["reasons"].append(reasons)
# add a point to the winner model
modelpoints[judge][winner_model] += 1
print(f"Done with match: {judge_counter}.{counter}")
print("Done with " + judge + " being a judge")
The collected data were manually annotated (Code reference), saved to a CSV file and uploaded as a dataset on HuggingFace hub.
2d. Post-Tournament Analysis
Code references: DebateLLMChampionship_analysis.ipynb and MotionCategoriesAssociations.ipynb
Post-tournament analysis involved:
- Analyzing words in motions and winning arguments when
QwQ-32B-Preview
was a judge
- Repeating the same analysis at 1. with
Llama-3.3-70B-Instruct
as a judge
- Repeating the same analysis at 1. with
Phi-3.5-mini-instruct
winning arguments
- Repeating the same analysis at 1. with with
HuggingFaceH4/starchat2-15b-v0.1
losing arguments
We also carried out topic association analysis for winning arguments with QwQ-32B-Preview
and Llama-3.3-70B-Instruct
as judges, as well as the same analysis for Phi-3.5-mini-instruct
winning arguments and HuggingFaceH4/starchat2-15b-v0.1
losing arguments.
These are the general functions defined for the analysis:
import pandas as pd
import nltk
from nltk.corpus import stopwords
from collections import Counter
import matplotlib.pyplot as plt
import seaborn as sns
from typing import Dict, List, Tuple
import numpy as np
df_qwq = df[df["judge"] == "Qwen/QwQ-32B-Preview"]
def compare_winning_arg_w_motion(df: pd.DataFrame) -> Dict:
"""
Analyzes the relationship between winning arguments and their motions.
Returns a dictionary containing analysis results and statistics.
"""
# Initialize containers for analysis
keyword_overlap_scores = []
winning_word_frequencies = Counter()
motion_word_frequencies = Counter()
favor_win_count = 0
against_win_count = 0
overlap_by_length = []
# Analysis results
results = {
'overlap_scores': [],
'word_frequencies': {},
'winning_sides': {},
'length_correlations': []
}
for index, row in df.iterrows():
motion = row["motions"]
motion_keywords = set(extract_keywords(motion))
motion_word_frequencies.update(motion_keywords)
# Determine winning argument
is_favor_winning = row["winner"] == row["favor_model"]
winning_arg = row["favor_arg"] if is_favor_winning else row["against_arg"]
# Update win counters
if is_favor_winning:
favor_win_count += 1
else:
against_win_count += 1
# Extract and analyze winning argument
common_words = set(extract_most_common_words(winning_arg, len(motion_keywords)))
winning_word_frequencies.update(common_words)
# Calculate overlap score
overlap = len(motion_keywords.intersection(common_words)) / len(motion_keywords)
keyword_overlap_scores.append(overlap)
# Record length correlation
overlap_by_length.append((len(winning_arg.split()), overlap))
# Store results
results['overlap_scores'] = keyword_overlap_scores
results['word_frequencies'] = {
'motion': dict(motion_word_frequencies.most_common(20)),
'winning_args': dict(winning_word_frequencies.most_common(20))
}
results['winning_sides'] = {
'favor': favor_win_count,
'against': against_win_count
}
results['length_correlations'] = overlap_by_length
# Create visualizations
create_analysis_plots(results)
return results
def create_analysis_plots(results: Dict):
"""Creates and displays analysis visualizations."""
# Set up the plotting area
plt.style.use('seaborn-v0_8-paper')
fig = plt.figure(figsize=(15, 10))
# 1. Overlap Score Distribution
plt.subplot(2, 2, 1)
sns.histplot(results['overlap_scores'], bins=20)
plt.title('Distribution of Keyword Overlap Scores')
plt.xlabel('Overlap Score')
plt.ylabel('Count')
# 2. Winning Sides Pie Chart
plt.subplot(2, 2, 2)
sides = results['winning_sides']
plt.pie([sides['favor'], sides['against']],
labels=['Favor', 'Against'],
autopct='%1.1f%%')
plt.title('Distribution of Winning Sides')
# 3. Word Frequencies Comparison
plt.subplot(2, 2, 3)
motion_words = list(results['word_frequencies']['motion'].keys())[:10]
motion_freqs = [results['word_frequencies']['motion'][w] for w in motion_words]
plt.barh(motion_words, motion_freqs)
plt.title('Top 10 Motion Keywords')
plt.xlabel('Frequency')
# 4. Length vs Overlap Scatter Plot
plt.subplot(2, 2, 4)
lengths, overlaps = zip(*results['length_correlations'])
plt.scatter(lengths, overlaps, alpha=0.5)
plt.title('Argument Length vs Keyword Overlap')
plt.xlabel('Argument Length (words)')
plt.ylabel('Overlap Score')
# Add trend line
z = np.polyfit(lengths, overlaps, 1)
p = np.poly1d(z)
plt.plot(lengths, p(lengths), "r--", alpha=0.8)
plt.tight_layout()
plt.show()
# Helper functions (assuming these exist)
def extract_keywords(text: str) -> List[str]:
"""Extract keywords from text. Implement your keyword extraction logic here."""
stop_words = set(stopwords.words('english'))
words = nltk.word_tokenize(text.lower())
return [w for w in words if w.isalnum() and w not in stop_words]
def extract_most_common_words(text: str, n: int) -> List[str]:
"""Extract n most common words from text."""
words = extract_keywords(text)
return [word for word, _ in Counter(words).most_common(n)]
3. Results and Conclusions
3a. Tournament Results
The tournament was won by Phi-3.5-mini-instruct
, with 5 overall victories and with being the winner also of the tournament batch in which Llama-3.3-70B-Instruct
was the judge (Fig 1).
It was followed, in the second place, by Mistral-7B-Instruct-v0.3
(4 victories, winner of the tournament batch in which QwQ-32B-Preview
was judge), Llama-3.1-8B-Instruct
(4 overall victories) and Qwen2.5-72B-Instruct
(4 overall victories).
In the third position we had starchat2-15b-v0.1
, with 2 overall victories.
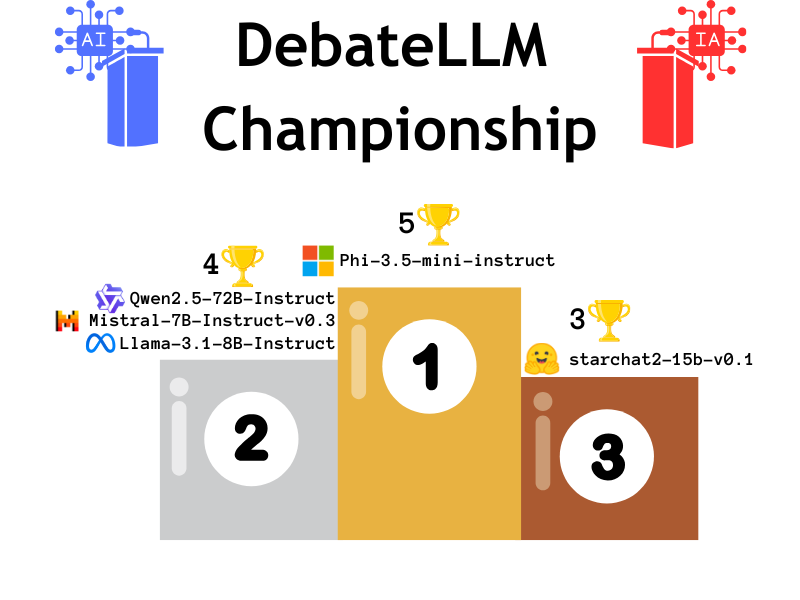
Fig 1: Tournament podium
3b. Favor and Against Winning Cases Distribution
Code reference: DebateLLMChampionship_analysis.ipynb
We first evaluated the “Favor” vs “Against” tendency for the two judges when deciding the winning arguments:
QwQ-32B-Preview
chose 5 times “Favor” and 5 times “Against”
Llama-3.3-70B-Instruct
chose 7 times “Favor” and 3 times “Against”
We repeated the same analysis for the cases in which Phi-3.5-mini-instruct
was the winner and for those in which starchat2-15b-v0.1
was the loser:
Phi-3.5-mini-instruct
won 3 time as “Favor” and 2 times as “Against”
starchat2-15b-v0.1
lost only when being “Against” the motion (and won twice while being in the “Favor” position and once while being “Against”)
3c. Overlapping between Key Words in Motions and Arguments
Code reference: DebateLLMChampionship_analysis.ipynb
We evaluated the overlapping score between the keywords in the motions and the keywords in the winning arguments in various settings:
- We evidenced broad variation of overlapping scores both with
QwQ-32B-Preview
and with Llama-3.3-70B-Instruct
as judges. Both the variation ranges were comparable, with the one in the winning arguments from Llama-3.3-70B-Instruct
being slightly narrower (Fig 2a-b)
- The overlapping scores for the winning prompts from
Phi-3.5-mini-instruct
were comparable with the ones registered for the previous point, but the variation was far broader than the one found for the losing prompts by starchat2-15b-v0.1
(Fig 2c-d)
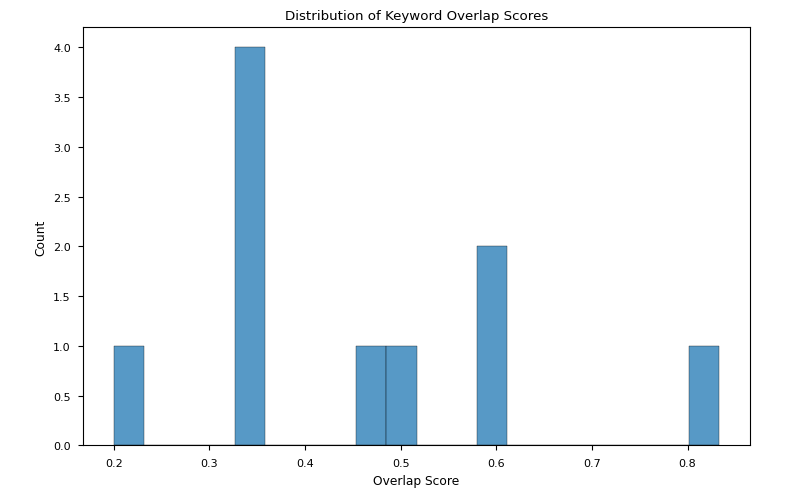
Fig 2a: Overlapping scores between the keywords in the motions and the keywords in the winning arguments distributions when QwQ-32B-Preview is a judge
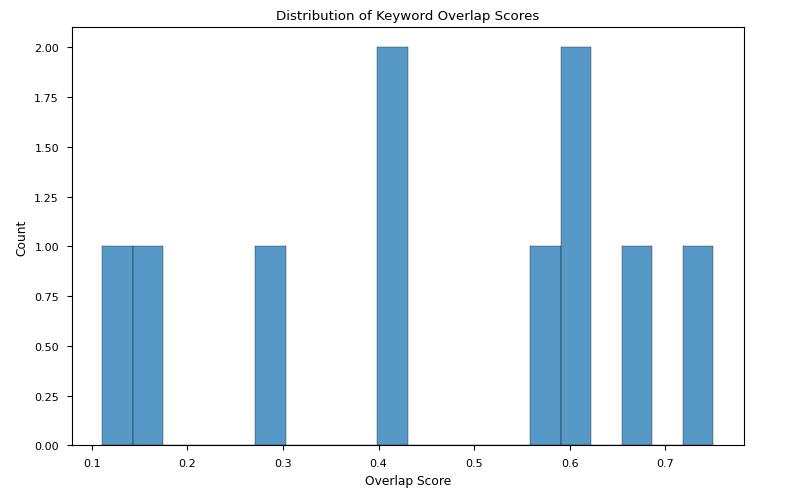
Fig 2b: Overlapping scores between the keywords in the motions and the keywords in the winning arguments distributions when Llama-3.3-70B-Instruct is a judge
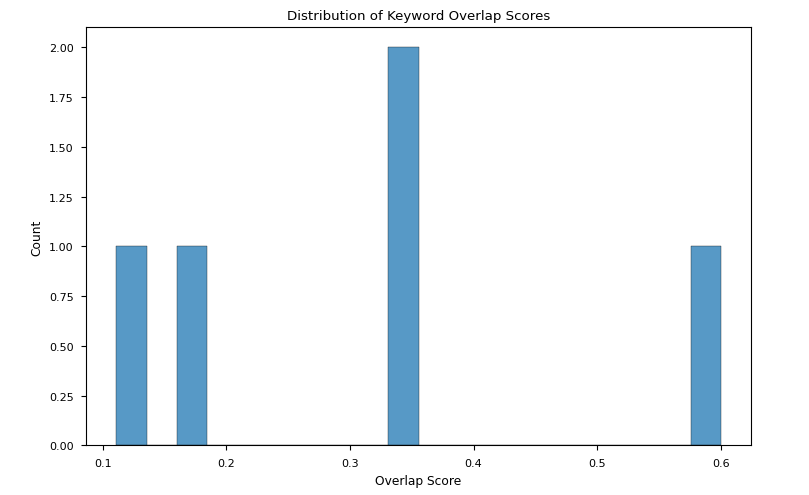
Fig 2c: Overlapping scores between the keywords in the motions and the keywords in the winning arguments distributions for winning arguments by Phi-3.5-mini-instruct
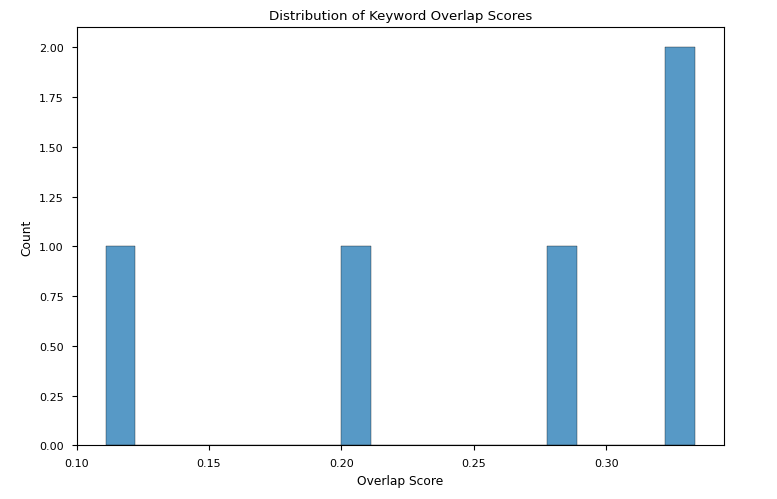
Fig 2d: Overlapping scores between the keywords in the motions and the keywords in the winning arguments distributions for losing arguments by starchat2-15b-v0.1
TAKEAWAY: Although results do not converge onto a single explanation, we could say that a high overlap score does not necessary help in winning, but that a low overlap score may have an influence on losing the match
We also evaluated the correlation among argument length (in words) and keyword overlapping score: while for overall winning arguments with both QwQ-32B-Preview
and Llama-3.3-70B-Instruct
as judges there is no significant correlation, Fig 3a-b highlight that there is a stronger positive correlation for Phi-3.5-mini-instruct
winning argument and a stronger negative correlation for starchat2-15b-v0.1
losing arguments.
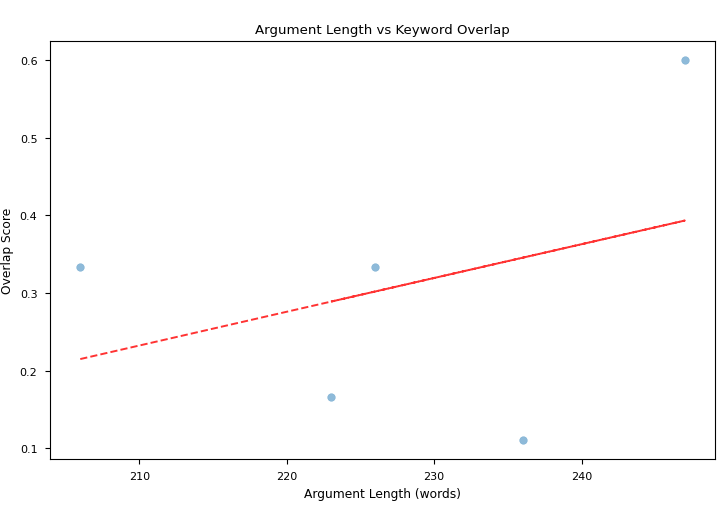
Fig 3a: Correlation between keyword overlapping scores and argument length for winning arguments by Phi-3.5-mini-instruct
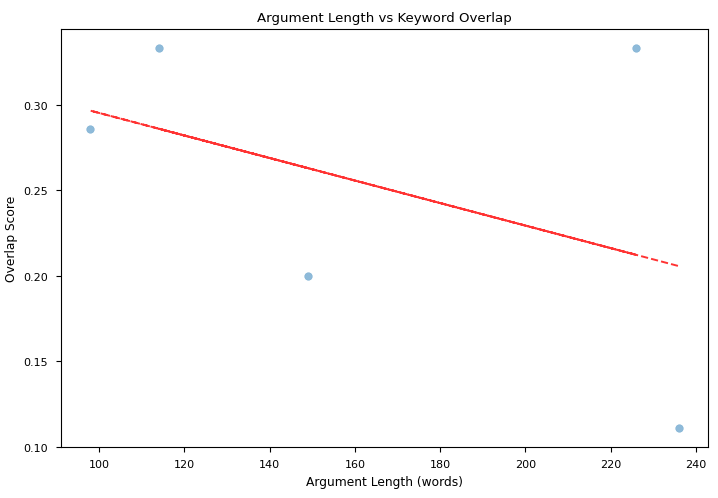
Fig 3b: Correlation between keyword overlapping scores and argument length for losing arguments by starchat2-15b-v0.1
TAKEAWAY: This correlation study might point at the fact that starchat2-15b-v0.1
was not able to maintain adherence to the original motion when producing longer arguments, and that might have lead to losing the matches. The ability of maintaining a broader correspondence to the original motion when producing longer arguments might, on the other hand, have influenced Phi-3.5-mini-instruct
victories.
3d. Motion Topics and Winning Arguments Correlation
Code reference: MotionCategoriesAssociations.ipynb
We lastly evaluated what positions (“Favor” or “Against”) were deemed winning in correlation to the topic of their motions.
First of all, we accounted for potential “personal opinion” influence (i.e. a bias in the LLM) in the choice of the winner, using gpt-4o-mini
to detect these biases and report them along with the expressions that contained “personal opinions” from the judge. We then build Table 1:
Judge |
Topic |
Position |
Influenced |
Quotes |
Qwen/QwQ-32B-Preview |
Prisoners Extradition |
Against |
False |
|
Qwen/QwQ-32B-Preview |
Oppose Chinese censorship |
Favor |
True |
The argument in favor is stronger because it emphasizes human rights, freedom of expression, and the need for a balanced approach to social stability. It aligns with international standards and promotes a more inclusive society. |
Qwen/QwQ-32B-Preview |
Democratization of UN |
Favor |
False |
|
Qwen/QwQ-32B-Preview |
Non-violent movements not leading social change |
Against |
False |
|
Qwen/QwQ-32B-Preview |
West funding a coup in Myanmar |
Against |
False |
|
Qwen/QwQ-32B-Preview |
Stop to Bullfighting |
Favor |
True |
The argument in favor of banning bullfighting is stronger due to its emphasis on ethical considerations. |
Qwen/QwQ-32B-Preview |
Paper is better than Internet |
Against |
False |
|
Qwen/QwQ-32B-Preview |
Ban to self-diagnose websites |
Favor |
True |
The potential for misdiagnosis and delayed treatment poses significant risks to public health. Privacy concerns further underscore the need for regulation or prohibition of these websites to ensure that individuals receive accurate and safe healthcare information and treatment. |
Qwen/QwQ-32B-Preview |
Public workers have right to strike |
Against |
False |
|
Qwen/QwQ-32B-Preview |
Hedge funds not purchasing sovereign debt |
Favor |
False |
|
meta-llama/Llama-3.3-70B-Instruct |
Trade Unions slow progress |
Favor |
False |
|
meta-llama/Llama-3.3-70B-Instruct |
Cancel 3rd World Debt |
Favor |
False |
|
meta-llama/Llama-3.3-70B-Instruct |
Deny terminally ill patients cures |
Against |
True |
the argument in favor was unable to present a coherent or convincing case. |
meta-llama/Llama-3.3-70B-Instruct |
Prioritized skilled refugees to enter EU |
Against |
True |
a humanitarian-focused approach is more aligned with principles of fairness and equality |
meta-llama/Llama-3.3-70B-Instruct |
Repatriate North Korean refugees |
Against |
True |
the moral and legal imperative to protect refugees’ lives and freedoms takes precedence. |
meta-llama/Llama-3.3-70B-Instruct |
Not replace workers with technology |
Favor |
False |
|
meta-llama/Llama-3.3-70B-Instruct |
Two parliaments: politicians and experts |
Favor |
True |
The argument in favor presents a more compelling case the benefits of integrating experts into the legislative process seem to outweigh the drawbacks. |
meta-llama/Llama-3.3-70B-Instruct |
Handmade gifts better than brand gifts |
Favor |
True |
The argument in favor presented a more compelling case highlighting the emotional value, personalization, and shared experiences that handmade gifts offer, which outweigh the potential drawbacks mentioned by the argument against. |
meta-llama/Llama-3.3-70B-Instruct |
Do not entrap pedophiles |
Favor |
False |
|
meta-llama/Llama-3.3-70B-Instruct |
Home-country trials for Guantanamo detainees |
Favor |
False |
|
Table 1: Potential influence of judge’s “personal opinion” in choosing the winner
Table 1 highlights that QwQ-32B-Preview
showed “personal opinion” influence in 30% of the cases, whereas Llama-3.3-70B-Instruct
in 50% of them: the difference might rely in the intrinsic reasoning structure that QwQ-32B-Preview
has, which might help avoiding bias-fed pitfalls in the judgement.
From Table 1 we can also see that both judges choose winning positions (except in few cases) that align with more liberal/left-leaning positions, which might be due to the political “bias” of LLMs, that all seem to align to libera/left-wing/social-democratic views (Rozado, 2024). To better asses the political leaning of our LLMs, we performed the political compass test on Llama-3.3-70B-Instruct
(judge), Phi-3.5-mini-instruct
and starchat2-15b-v0.1
(the winner and the loser of the tournament) (Fig 4).
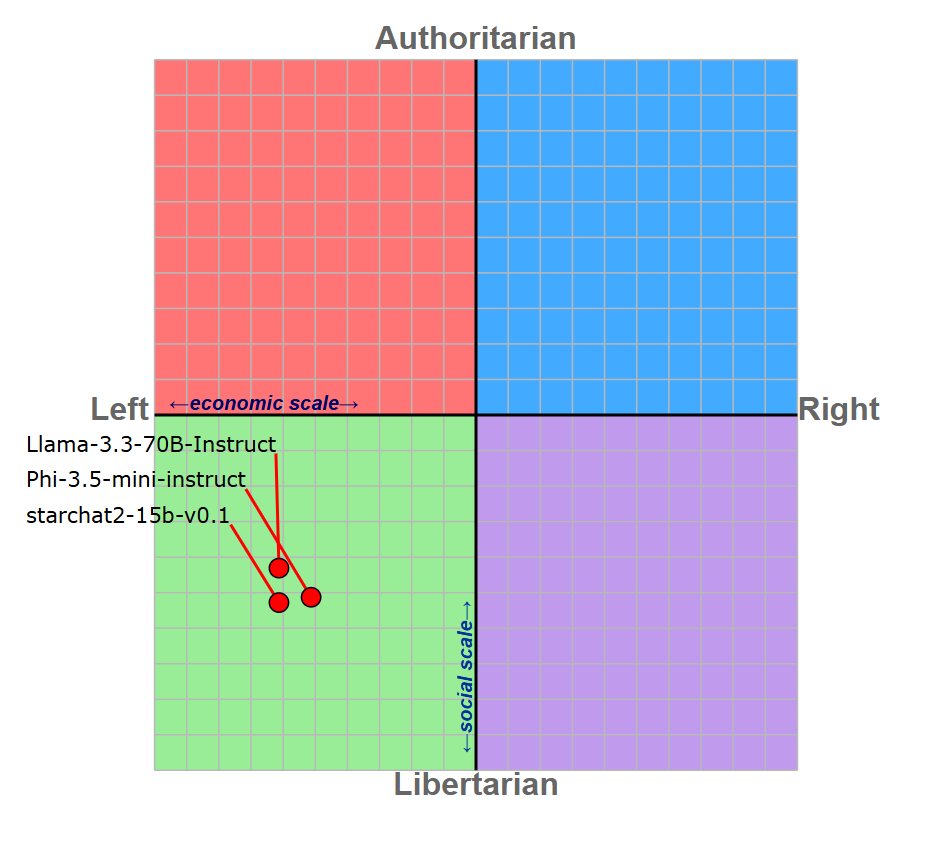
Fig 4: Political compass of the three evaluated LLMs
The political compass gives insight on left-leaning, libertarian positions for the three evaluated LLMs: this might mean that the judges positions in the choice of the were influenced by an internal political bias. The intrinsic political leaning of the models may have influenced also the winning chances for Phi-3.5-mini-instruct
and starchat2-15b-v0.1
(Table 2):
Model |
Position |
Topics |
microsoft/Phi-3.5-mini-instruct (winning) |
Against |
West funding a coup in Myanmar, Repatriate North Korean refugees |
microsoft/Phi-3.5-mini-instruct (winning) |
Favor |
Ban to self-diagnose websites, Handmade gifts better than brand gifts, Do not entrap pedophiles |
HuggingFaceH4/starchat2-15b-v0.1 (losing) |
Against |
Democratization of UN, Stop to Bullfighting, Ban to self-diagnose websites, Not replace workers with technology, Handmade gifts better than brand gifts |
HuggingFaceH4/starchat2-15b-v0.1 (losing) |
Favor |
None |
As you can see, starchat2-15b-v0.1
needed to defend the position against several issues that are generally supported by liberal/left-wing political views: in this sense, the model might have hard a hard time generating a valid argument.
On the other side, all the positions that Phi-3.5-mini-instruct
had to defend were aligned with its political views, making it easier for thr LLM to generate convincing and winning arguments.
TAKEAWAY: There might be a correlation between the political leanings of the LLMs and their preferences in winner choice/ability to generate convincing arguments
4. Data and Code Availability
The code is available for reproduction as AstraBert/DebateLLM-Championship GitHub repo. The code is structured as three Google Colab notebooks that execute the code reported in this blog post.
The collected debate data are available as as-cle-bert/DebateLLMs on HuggingFace Hub.